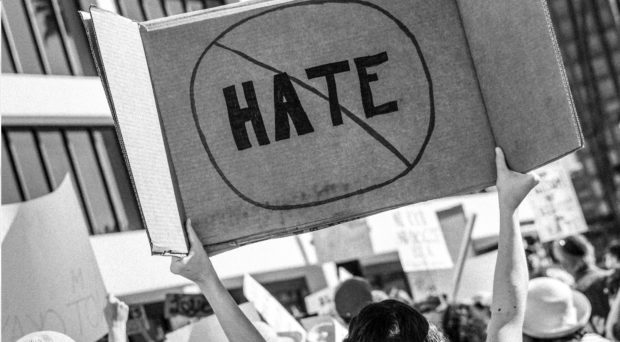
In an attempt to understand which factors cause crime to cluster in particular places at specific times, environmental criminologists have shifted the focus of their analysis from the individual who commits or suffers the crime to the environment where it occurs. Their starting premise is that the characteristics of each environment favor or hinder crime and that it is possible to intervene in these environments to control it.
Hate speech is a low-prevalence phenomenon and is difficult to detect amid all the noise. It is complex to define and it is an extraordinarily adaptive phenomenon
The Cybercrime and Place theoretical framework has recently been developed to extrapolate the analysis of crime places into cyberspace. Certainly, it seems counter-intuitive to talk about places in cyberspace, but the connotation that environmental criminology gives to the concept of place goes beyond the physical space. What counts about these cyber places is that they allow convergence of people and things. And, in the absence of guardians, this convergence generates opportunities to commit crimes.
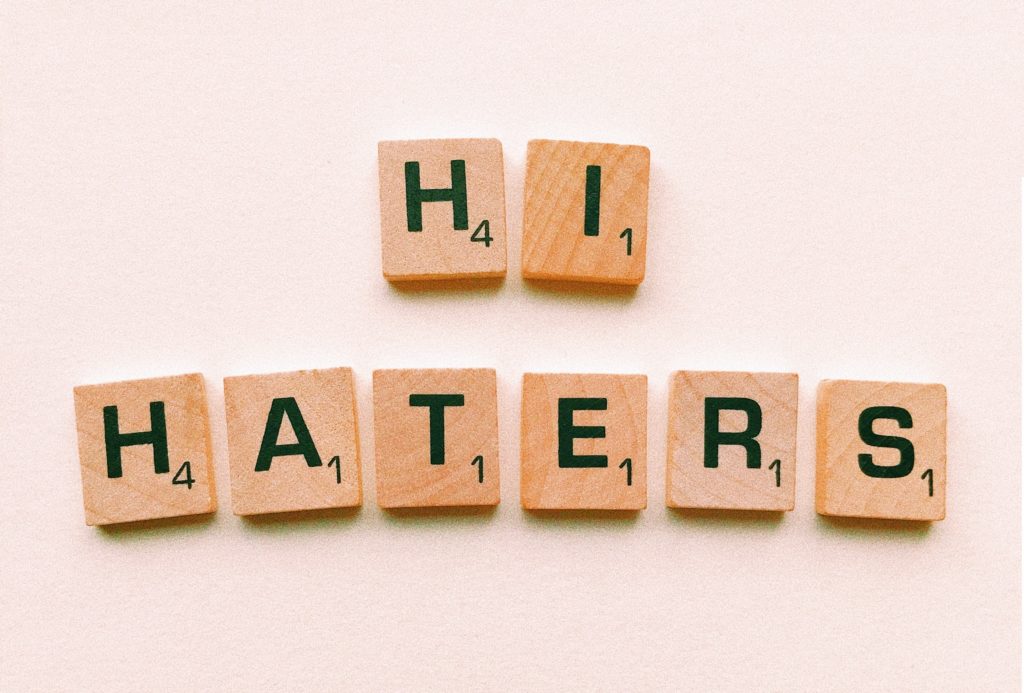
Twitter and hate speech
On Twitter, users constantly engage with information posted by other users, at the micro level, in digital microenvironments, defined by the combination of the people (i.e., accounts), who say things (i.e., tweets) to other people (i.e., other accounts). Most of the time this is a harmless activity, but it is possible that one of these tweets contains a type of radical content called hate speech.
Hundreds of millions of tweets are posted daily on Twitter. Police and service providers screen Twitter every day looking for hate speech in order to remove it. But hate speech is a low-prevalence phenomenon and is difficult to detect amid all the noise. It is unrealistic to think they can control all the content posted on Twitter. Yet, users expect them to do so.
We have developed a machine learning model that feeds on the metadata of each tweet to determine whether it contains hate speech with a 92% precision.
Hate speech detection poses two main challenges. First, it is complex to define and therefore to delimit. Secondly, the dynamism of language converts it into an extraordinarily adaptive phenomenon. Traditionally semantic approaches have been used for its detection. These approaches consider a message to be hate speech if it contains certain words previously classified as radical. But depending on the context some words can be misleading and cause incorrect classification.
We have used an alternative approach to overcome the limitations of these approaches. In our paper we hypothesize that the characteristics of digital microenvironments condition hate speech patterns. Based on this idea, we have developed a machine learning model that feeds on the metadata of each tweet to determine whether it contains hate speech with a 92% precision. By using a sample of tweets sent via Twitter following the June 2017 London Bridge terror attack (N = 200,880), the present study introduces a new algorithm designed to detect hate speech messages in cyberspace.
Through the application of the machine learning classification technique Random Forests, our analysis indicates that metadata associated with the interaction and structure of tweets are especially relevant to identify the content they contain. We thus expect to facilitate and reduce the analysis tasks performed by police and service providers to mitigate the impact of hate speech on social network users.
Comments